ORCID
0000-0002-4299-570X (Thomadakis), 0000-0002-8433-2234 (Billias), 0000-0003-3088-0187 (Chrisochoides)
College
The Graduate School
Department
Computer Science
Graduate Level
Master’s
Publication Date
2023
DOI
10.25883/qa2r-rw96
Abstract
Future particle accelerators will exceed by far the current data size (1015) per experiment, and high- luminosity program(s) will produce more than 300 times as much data. Classical Machine Learning (ML) likely will benefit from new tools based on quantum computing. Particle track reconstruction is the most computationally intensive process in nuclear physics experiments. A combinatorial approach exhaustively tests track measurements (“hits”), represented as images, to identify those that form an actual particle trajectory, which is then used to reconstruct track parameters necessary for the physics experiment. Quantum Machine Learning (QML) could improve this process in multiple ways, including denoising the data, classifying candidate tracks, and reconstructing the track parameters without conventional processing.
We will present our contributions to the candidate track classification problem using a quantum convolutional network, for Noisy Intermediate-Scale Quantum (NISQ) computers: (1) an artifact-free image decomposition of the particle images into sub-images to cope with the 5% fidelity of a 12-qubit circuit required for the encoding of the full image (currently used in classical ML); (2) improve fidelity by 70% utilizing Distributed NISQ (D-NSIQ) model that utilizes 128 cores to run our simulations on 5-qubit Quantum Processor Units with different and realistic noise models; (3) evaluation of D-NISQ QML in terms of accuracy against the 99% precision of an optimized classical convolutional network we published recently.
Disciplines
Artificial Intelligence and Robotics | Quantum Physics | Software Engineering
Files
Download Full Text (675 KB)
Recommended Citation
Thomadakis, Polykarpos; Billias, Emmanuel; and Chrisochoides, Nikos, "Evaluation of Scalable Quantum and Classical Machine Learning for Particle Tracking Classification in Nuclear Physics" (2023). The Graduate School Posters. 3.
https://digitalcommons.odu.edu/gradposters2023_gradschool/3
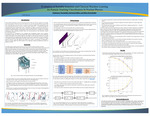
Included in
Artificial Intelligence and Robotics Commons, Quantum Physics Commons, Software Engineering Commons