ORCID
0000-0002-6578-4657 (Witherow)
College
Frank Batten College of Engineering and Technology
Department
Electrical/Computer Engineering
Program
PhD Electrical & Computer Engineering
Publication Date
4-2021
DOI
10.25883/yyt7-6q42
Abstract
While state-of-the-art deep learning models have demonstrated success in adult facial expression classification by leveraging large, labeled datasets, labeled data for child facial expression classification is limited. Due to differences in facial morphology and development in child and adult faces, deep learning models trained on adult data do not generalize well to child data. Recent deep domain adaptation approaches have improved the generalizability of models trained on a source domain to a target domain with few labeled samples. We propose that incorporating steps of deep transfer learning, e.g. weights initialization from the pre-trained source model and freezing model layers, may improve the domain adaptation. Knowledge of a few labeled child (target domain) examples is incorporated into the adult data distribution (source domain) by training a Siamese architecture with pairs of labeled source and target images. A contrastive semantic alignment (CSA) loss is then used to align the source and target representations to learn a domain-invariant latent representation. In this work, deep transfer learning and domain adaptation approaches are combined to adapt the source (adult facial expression) model for seven class (‘anger’, ‘disgust’, ‘fear’, ‘happy’, ‘sad’, ‘surprise’, plus ‘neutral’) child facial expression (target) classification, using 10 or fewer target samples per expression. Using only 10 samples per expression class, our hybrid approach exceeds the performance of the transfer learning model by more than 12% on mean accuracy over ten cross validation folds.
Disciplines
Computer Sciences | Data Science | Electrical and Computer Engineering
Files
Download Full Text (1.1 MB)
Recommended Citation
Witherow, Megan; Shields, Winston; Samad, Manar; and Iftekharuddin, Khan, "Data-Limited Domain Adaptation and Transfer Learning for Learning Latent Expression Labels of Child Facial Expression Images" (2021). College of Engineering & Technology (Batten) Posters. 3.
https://digitalcommons.odu.edu/gradposters2021_engineering/3
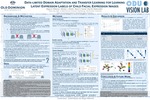
Included in
Computer Sciences Commons, Data Science Commons, Electrical and Computer Engineering Commons