Document Type
Article
Publication Date
2025
DOI
10.3390/ijms26041707
Publication Title
International Journal of Molecular Sciences
Volume
26
Issue
4
Pages
1707 (1-18)
Abstract
The rapid growth of diverse -omics datasets has made multiomics data integration crucial in cancer research. This study adapts the expectation–maximization routine for the joint latent variable modeling of multiomics patient profiles. By combining this approach with traditional biological feature selection methods, this study optimizes latent distribution, enabling efficient patient clustering from well-studied cancer types with reduced computational expense. The proposed optimization subroutines enhance survival analysis and improve runtime performance. This article presents a framework for distinguishing cancer subtypes and identifying potential biomarkers for breast cancer. Key insights into individual subtype expression and function were obtained through differentially expressed gene analysis and pathway enrichment for BRCA patients. The analysis compared 302 tumor samples to 113 normal samples across 60,660 genes. The highly upregulated gene COL10A1, promoting breast cancer progression and poor prognosis, and the consistently downregulated gene CDG300LG, linked to brain metastatic cancer, were identified. Pathway enrichment analysis revealed similarities in cellular matrix organization pathways across subtypes, with notable differences in functions like cell proliferation regulation and endocytosis by host cells. GO Semantic Similarity analysis quantified gene relationships in each subtype, identifying potential biomarkers like MATN2, similar to COL10A1. These insights suggest deeper relationships within clusters and highlight personalized treatment potential based on subtypes.
Rights
© 2025 by the authors.
This article is an open access article distributed under the terms and conditions of the Creative Commons Attribution 4.0 International (CC BY 4.0) license.
Data Availability
Article states: "Data are contained within the article."
Original Publication Citation
Pateras, J., Lodi, M., Rana, P., & Ghosh, P. (2025). Heterogeneous clustering of multiomics data for breast cancer subgroup classification and detection. International Journal of Molecular Sciences, 26(4), 1-18, Article 1707. https://doi.org/10.3390/ijms26041707
Repository Citation
Pateras, J., Lodi, M., Rana, P., & Ghosh, P. (2025). Heterogeneous clustering of multiomics data for breast cancer subgroup classification and detection. International Journal of Molecular Sciences, 26(4), 1-18, Article 1707. https://doi.org/10.3390/ijms26041707
ORCID
0000-0001-9199-2479 (Rana)
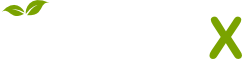
- Usage
- Downloads: 4
- Abstract Views: 1
- Captures
- Readers: 1
- Mentions
- Blog Mentions: 1
- News Mentions: 1