Document Type
Article
Publication Date
2016
DOI
10.1016/j.dcan.2016.05.002
Publication Title
Digital Communications and Networks
Volume
2
Issue
3
Pages
97-107
Abstract
This work is dedicated to constructing a multi-scale structural health monitoring system to monitor and evaluate the serviceability of bridges based on the Hadoop Ecosystem (MS-SHM-Hadoop). By taking the advantages of the fault-tolerant distributed file system called the Hadoop Distributed File System (HDFS) and high-performance parallel data processing engine called MapReduce programming paradigm, MS-SHM-Hadoop features include high scalability and robustness in data ingestion, fusion, processing, retrieval, and analytics. MS-SHM-Hadoop is a multi-scale reliability analysis framework, which ranges from nationwide bridge-surveys, global structural integrity analysis, and structural component reliability analysis. This Nationwide bridge survey uses deep-learning techniques to evaluate the bridge serviceability according to real-time sensory data or archived bridge-related data such as traffic status, weather conditions and bridge structural configuration. The global structural integrity analysis of a targeted bridge is made by processing and analyzing the measured vibration signals incurred by external loads such as wind and traffic flow. Component-wise reliability analysis is also enabled by the deep learning technique, where the input data is derived from the measured structural load effects, hyper-spectral images, and moisture measurement of the structural components. As one of its major contributions, this work employs a Bayesian network to formulate the integral serviceability of a bridge according to its components serviceability and inter-component correlations. Here the inter-component correlations are jointly specified using a statistics-oriented machine learning method (e.g., association rule learning) or structural mechanics modeling and simulation.
Original Publication Citation
Liang, Y., Wu, D., Liu, G., Li, Y., Gao, C., Ma, Z. J., & Wu, W. (2016). Big data-enabled multiscale serviceability analysis for aging bridges. Digital Communications and Networks, 2(3), 97-107. doi: 10.1016/j.dcan.2016.05.002
Repository Citation
Liang, Y., Wu, D., Liu, G., Li, Y., Gao, C., Ma, Z. J., & Wu, W. (2016). Big data-enabled multiscale serviceability analysis for aging bridges. Digital Communications and Networks, 2(3), 97-107. doi: 10.1016/j.dcan.2016.05.002
ORCID
0000-0003-0178-1876 (Yaohang Li), 0000-0002-3019-1886 (Cuilan Gao), 0000-0001-8246-7605 (Zhongguo John Ma)
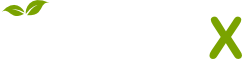
- Citations
- Citation Indexes: 42
- Policy Citations: 1
- Usage
- Downloads: 125
- Abstract Views: 35
- Captures
- Readers: 138
Comments
This work is jointly sponsored by the National Science Foundation (NSF) with proposal number 1240734 and the UTC THEC/CEACSE 2016 Grant Program.
Open Access funded by Chongquing University of Posts and Telecommunications, published under an Attribution-Noncommercial-NoDerivatives 4.0 International (CC BY-NC-ND-4.0) license (https://creativecommons.org/licenses/by-nc-nd/4.0/).