Document Type
Article
Publication Date
2020
DOI
10.3390/rs12152455
Publication Title
Remote Sensing
Volume
12
Issue
15
Pages
17 pp.
Abstract
Natural disasters such as flooding can severely affect human life and property. To provide rescue through an emergency response team, we need an accurate flooding assessment of the affected area after the event. Traditionally, it requires a lot of human resources to obtain an accurate estimation of a flooded area. In this paper, we compared several traditional machine-learning approaches for flood detection including multi-layer perceptron (MLP), support vector machine (SVM), deep convolutional neural network (DCNN) with recent domain adaptation-based approaches, based on a multi-modal and multi-temporal image dataset. Specifically, we used SPOT-5 and RADAR images from the flood event that occurred in November 2000 in Gloucester, UK. Experimental results show that the domain adaptation-based approach, semi-supervised domain adaptation (SSDA) with 20 labeled data samples, achieved slightly better values of the area under the precision-recall (PR) curve (AUC) of 0.9173 and F1 score of 0.8846 than those by traditional machine approaches. However, SSDA required much less labor for ground-truth labeling and should be recommended in practice.
Original Publication Citation
Islam, K. A., Uddin, M. S., Kwan, C., & Li, J. (2020). Flood detection using multi-modal and multi-temporal images: A comparative study. Remote Sensing, 12(15), 17 pp., Article 2455. https://doi.org/10.3390/rs12152455
Repository Citation
Islam, Kazi Aminul; Uddin, Mohammad Shahab; Kwan, Chiman; and Li, Jiang, "Flood Detection Using Multi-Modal and Multi-Temporal Images: A Comparative Study" (2020). Electrical & Computer Engineering Faculty Publications. 268.
https://digitalcommons.odu.edu/ece_fac_pubs/268
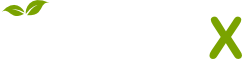
- Citations
- Citation Indexes: 41
- Policy Citations: 1
- Usage
- Downloads: 177
- Abstract Views: 18
- Captures
- Readers: 51
Included in
Electrical and Computer Engineering Commons, Other Oceanography and Atmospheric Sciences and Meteorology Commons
Comments
© 2020 by the Authors. This article is an open access article distributed under the terms and conditions of the Creative Commons Attribution (CC BY) license.