Document Type
Article
Publication Date
2020
DOI
10.1103/PhysRevAccelBeams.23.114601
Publication Title
Physical Review Accelerators and Beams
Volume
23
Issue
11
Pages
114601 (1-16)
Abstract
We report on the development of machine learning models for classifying C100 superconducting radio-frequency (SRF) cavity faults in the Continuous Electron Beam Accelerator Facility (CEBAF) at Jefferson Lab. CEBAF is a continuous-wave recirculating linac utilizing 418 SRF cavities to accelerate electrons up to 12 GeV through five passes. Of these, 96 cavities (12 cryomodules) are designed with a digital low-level rf system configured such that a cavity fault triggers waveform recordings of 17 rf signals for each of the eight cavities in the cryomodule. Subject matter experts are able to analyze the collected time-series data and identify which of the eight cavities faulted first and classify the type of fault. This information is used to find trends and strategically deploy mitigations to problematic cryomodules. However, manually labeling the data is laborious and time consuming. By leveraging machine learning, near real-time—rather than postmortem—identification of the offending cavity and classification of the fault type has been implemented. We discuss performance of the machine learning models during a recent physics run. Results show the cavity identification and fault classification models have accuracies of 84.9% and 78.2%, respectively.
Original Publication Citation
Tennant, C., Carpenter, A., Powers, T., Shabalina Solopova, A., Vidyaratne, L., & Iftekharuddin, K. (2020). Superconducting radio-frequency cavity fault classification using machine learning at Jefferson Laboratory. Physical Review Accelerators and Beams, 23(11), 1-16, Article 114601. https://doi.org/10.1103/PhysRevAccelBeams.23.114601
Repository Citation
Tennant, Chris; Carpenter, Adam; Powers, Tom; Solopova, Anna Shabalina; Vidyaratne, Lasitha; and Iftekharuddin, Khan, "Superconducting Radio-Frequency Cavity Fault Classification Using Machine Learning at Jefferson Laboratory" (2020). Electrical & Computer Engineering Faculty Publications. 283.
https://digitalcommons.odu.edu/ece_fac_pubs/283
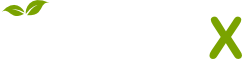
- Citations
- Citation Indexes: 44
- Usage
- Downloads: 79
- Abstract Views: 4
- Captures
- Readers: 29
- Mentions
- Blog Mentions: 1
- News Mentions: 4
Comments
Published by the American Physical Society under the terms of the Creative Commons Attribution 4.0 International license. Further distribution of this work must maintain attribution to the author(s) and the published article's title, journal citation, and DOI.