ORCID
0000-0002-1476-113X (Chakraborty), 0000-0002-8991-1737 (Pant)
Document Type
Article
Publication Date
Spring 2-12-2025
DOI
10.3390/computation13020051
Publication Title
Computation
Volume
13
Issue
2
Pages
51
Abstract
Introduction: In the field of medical research, empirical Bayesian analysis has emerged as an increasingly applicable approach. This statistical framework offers greater flexibility, enabling researchers to incorporate prior information and rigorously estimate parameters of interest. However, the selection of suitable prior distributions can be a challenging endeavor, with profound implications for the resulting inferences. To address this challenge, this study proposes a new analytical procedure that leverages resampling techniques to guide the choice of priors in Bayesian analysis. Subject and Methods: The study group consisted of patients who had been diagnosed and had died of pancreatic adenocarcinoma (cause-specific death) who had undergone both chemotherapy and radiation at stage IV of cancer. The data were collected from the Surveillance, Epidemiology, and End Results (SEER) database. Initially, the most suitable probabilistic behavior of the survival times of patients was identified parametrically via goodness-of-fit (GOF) tests, and afterward, empirical Bayesian analysis (EBA) was performed using resampling techniques (bootstrapping and the jackknife method). The Hamiltonian Monte Carlo (HMC) method was used to obtain the posterior distribution. Results: The most appropriate data distribution was found to be a two-parameter log-normal via GOF tests. A sensitivity analysis, followed by a simulation study, was performed to validate the analytical method. The performance of bootstrapped and jackknifed empirical Bayesian estimates was compared with maximum likelihood (ML) methods at each simulation stage. The empirical Bayesian estimates were found to be consistent with the ML estimates. Finally, a comparison was made among the parametric, Kaplan–Meier and empirical Bayesian survival estimates at different time points to illustrate the validity of the method. Conclusions: Determining the appropriate prior distribution is one of the crucial components in Bayesian analysis, as it can significantly influence the resulting inferences. The cautious selection of the prior information is essential, as it encapsulates the researcher’s beliefs or external prior knowledge about the parameters of interest. In the Bayesian framework, empirical resampling methods, such as bootstrapping and jackknifing, can offer valuable insights into the significance of prior selection, thus improving the consistency of statistical inferences. However, the analytical procedure is based on the time-to-event data, and the prior selection procedure can be extended to any real data, where Bayesian analysis is needed for decision-making and uncertainty quantification.
Rights
© 2025 by the authors. Licensee MDPI, Basel, Switzerland.
This article is an open access article distributed under the terms and conditions of the Creative Commons Attribution (CC BY) license (https://creativecommons.org/licenses/by/4.0/).
Data Availability
Data can be extracted from https://seer.cancer.gov/seerstat/ (accessed on 28 February 2021)
Original Publication Citation
Chakraborty, A.; Pant, M.D. (2025). An analytical prior selection procedure for empirical Bayesian analysis using resampling techniques: A simulation-based approach using the pancreatic adenocarcinoma data from the SEER database. Computation, 13(2), Article 51. https://doi.org/10.3390/computation13020051
Repository Citation
Chakraborty, A.; Pant, M.D. (2025). An analytical prior selection procedure for empirical Bayesian analysis using resampling techniques: A simulation-based approach using the pancreatic adenocarcinoma data from the SEER database. Computation, 13(2), Article 51. https://doi.org/10.3390/computation13020051
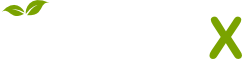
- Usage
- Downloads: 3
- Abstract Views: 2
- Captures
- Readers: 1
- Mentions
- Blog Mentions: 1
- News Mentions: 1
Included in
Other Statistics and Probability Commons, Statistical Methodology Commons, Survival Analysis Commons