College
College of Sciences
Program
Ph.D. Computational and Applied Mathematics -- Mathematics and Statistics
Publication Date
Spring 2020
DOI
10.25883/wspk-kp13
Abstract
High-dimensional dependent binary data are prevalent in a wide range of scientific disciplines. A popular method for analyzing such data is the Multivariate Probit (MP) model. But the MP model sometimes fails even within a feasible range of binary correlations, because the underlying correlation matrix of the latent variables may not be positive definite. In this research, we proposed pair copula models, assuming the dependence between the binary variables is first order autoregressive (AR(1))or equicorrelated structure. Also, when Archimediean copula is used, most paper converted Kendall Tau to corresponding copula parameter, there is no explicit function of Pearson’s correlation coefficient with copula parameter. Therefore, we obtain the relationship between binary variable coefficient with copula parameter in the study as well. The outline of this poster presentation is as follows: we start with the definition of the copula and pictorially illustrate the relation between the copula parameter and the binary correlation. We illustrate pair copula constructions of multivariate binary distributions using D-vines and C-vines. We show the application of our method on a real life data. Finally, we briefly discuss our ongoing research.
Keywords
Binary data, Multivariate Probit (MP) model, D-vine, Copula parameter, Coefficient
Disciplines
Applied Statistics | Numerical Analysis and Computation | Probability
Files
Download Full Text (577 KB)
Recommended Citation
Lin, Huihui and Chaganty, N. Rao, "D-Vine Copula Model For Dependent Binary Data" (2020). College of Sciences Posters. 4.
https://digitalcommons.odu.edu/gradposters2020_sciences/4
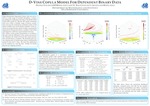
Included in
Applied Statistics Commons, Numerical Analysis and Computation Commons, Probability Commons