ORCID
0000-0002-9318-8844 (Choudhury), 0000-0002-6162-2896 (Salsabil), 0000-0003-4748-9176 (Jayanetti), 0000-0003-0173-4463 (Wu)
College
College of Sciences
Department
Computer Science
Graduate Level
Doctoral
Publication Date
2023
DOI
10.25883/a3qt-j935
Abstract
Metadata quality is crucial for digital objects to be discovered through digital library interfaces. Although DL systems have adopted Dublin Core to standardize metadata formats (e.g., ETD-MS v1.11), the metadata of digital objects may contain incomplete, inconsistent, and incorrect values [1]. Most existing frameworks to improve metadata quality rely on crowdsourced correction approaches, e.g., [2]. Such methods are usually slow and biased toward documents that are more discoverable by users. Artificial intelligence (AI) based methods can be adopted to overcome this limit by automatically detecting, correcting, and canonicalizing the metadata, featuring quick and unbiased responses to document metadata. This paper uses Electronic Theses and Dissertations (ETDs) metadata as a case study and proposes an AI-based framework to improve metadata quality.
ETD represents scholarly works of students who pursue higher education and successfully meet the partial requirement of a degree. ETDs are usually hosted by university libraries or ProQuest. Using web crawling techniques, we collected metadata and full text of 533,047 ETDs from 114 American universities. Upon inspecting the metadata of these ETDs, we noticed many ETD repositories are accompanied by incomplete, inconsistent, or incorrect metadata. We propose MetaEnhance, a framework that utilizes state-of-the-art AI methods to improve the quality of seven key metadata fields, including title, author, university, year, degree, advisor, and department. To evaluate MetaEnhance, we compiled a benchmark containing 500 ETDs, by combining subsets sampled using different criteria. We evaluated MetaEnhance against this benchmark and found that the proposed methods achieved remarkable performance in detecting and correcting metadata errors.
Keywords
Digital libraries, Scholarly big data, ETD, Metadata quality
Disciplines
Artificial Intelligence and Robotics | Data Science | Other Computer Sciences
Files
Recommended Citation
Choudhury, Muntabir H.; Salsabil, Lamia; Jayanetti, Himarsha R.; and Wu, Jian, "MetaEnhance: Metadata Quality Improvement for Electronic Theses and Dissertations" (2023). College of Sciences Posters. 5.
https://digitalcommons.odu.edu/gradposters2023_sciences/5
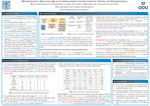
Included in
Artificial Intelligence and Robotics Commons, Data Science Commons, Other Computer Sciences Commons
Comments
References:
[1] Yen Bui and Jung-ran Park. 2013. An Assessment of Metadata Quality: A Case Study of the National Science Digital Library Metadata Repository. Proceedings of the Annual Conference of CAIS / Actes du congr`es annuel de l’ACSI (Oct. 2013). https://doi.org/10.29173/cais166
[2] Jian Wu, Kyle Williams, Madian Khabsa, and C.L. Giles. 2015. The Impact of User Corrections On A Crawl-Based Digital Library: A CiteSeerX Perspective. (01 2015), 171–176. https://doi.org/10.4108/icst.collaboratecom.2014.257563
Footnote:
1 https://ndltd.org/wp-content/uploads/2021/04/etd-ms-v1.1.html