Document Type
Article
Publication Date
2023
DOI
10.1137/22M147236X
Publication Title
SIAM/ASA Journal on Uncertainty Quantification
Volume
11
Issue
1
Pages
262-284
Abstract
Image reconstruction based on indirect, noisy, or incomplete data remains an important yet challenging task. While methods such as compressive sensing have demonstrated high-resolution image recovery in various settings, there remain issues of robustness due to parameter tuning. Moreover, since the recovery is limited to a point estimate, it is impossible to quantify the uncertainty, which is often desirable. Due to these inherent limitations, a sparse Bayesian learning approach is sometimes adopted to recover a posterior distribution of the unknown. Sparse Bayesian learning assumes that some linear transformation of the unknown is sparse. However, most of the methods developed are tailored to specific problems, with particular forward models and priors. Here, we present a generalized approach to sparse Bayesian learning. It has the advantage that it can be used for various types of data acquisitions and prior information. Some preliminary results on image reconstruction/recovery indicate its potential use for denoising, deblurring, and magnetic resonance imaging.
Rights
© 2023 Society for Industrial and Applied Mathematics and American Statistical Association. Unauthorized reproduction of this article is prohibited.
"The Author may post the final published version of the Work on the Author's personal web site and on the web server of the Author's institution, provided that proper notice of the Publisher's copyright is included and that no separate or additional fees are collected for access to or distribution of the work."
Original Publication Citation
Glaubitz, J., Gelb, A., & Song, G. (2023). Generalized sparse Bayesian learning and application to image reconstruction. SIAM/ASA Journal on Uncertainty Quantification, 11(1), 262-284. https://doi.org/10.1137/22M147236X
Repository Citation
Glaubitz, Jan; Gelb, Anne; and Song, Guohui, "Generalized Sparse Bayesian Learning and Application to Image Reconstruction" (2023). Mathematics & Statistics Faculty Publications. 230.
https://digitalcommons.odu.edu/mathstat_fac_pubs/230
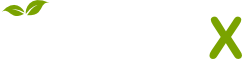
- Citations
- Citation Indexes: 12
- Usage
- Downloads: 116
- Abstract Views: 5
- Captures
- Readers: 6
- Mentions
- News Mentions: 1