Document Type
Article
Publication Date
2024
DOI
10.3390/diagnostics14161699
Publication Title
Diagnostics
Volume
14
Issue
16
Pages
1699 (1-17)
Abstract
The class activation map (CAM) represents the neural-network-derived region of interest, which can help clarify the mechanism of the convolutional neural network’s determination of any class of interest. In medical imaging, it can help medical practitioners diagnose diseases like COVID-19 or pneumonia by highlighting the suspicious regions in Computational Tomography (CT) or chest X-ray (CXR) film. Many contemporary deep learning techniques only focus on COVID-19 classification tasks using CXRs, while few attempt to make it explainable with a saliency map. To fill this research gap, we first propose a VGG-16-architecture-based deep learning approach in combination with image enhancement, segmentation-based region of interest (ROI) cropping, and data augmentation steps to enhance classification accuracy. Later, a multi-layer Gradient CAM (ML-Grad-CAM) algorithm is integrated to generate a class-specific saliency map for improved visualization in CXR images. We also define and calculate a Severity Assessment Index (SAI) from the saliency map to quantitatively measure infection severity. The trained model achieved an accuracy score of 96.44% for the three-class CXR classification task, i.e., COVID-19, pneumonia, and normal (healthy patients), outperforming many existing techniques in the literature. The saliency maps generated from the proposed ML-GRAD-CAM algorithm are compared with the original Gran-CAM algorithm.
Rights
© 2024 The Authors.
This article is an open access article distributed under the terms and conditions of the Creative Commons Attribution 4.0 International (CC BY 4.0) License.
Data Availability
Article states: "The dataset is publicly available at https://www.kaggle.com/datasets/tawsifurrahman/covid19-radiography-database accessed on 22 March 2022, and the source code will be available upon request."
Original Publication Citation
Rahman, M. F., Tseng, T. B., Pokojovy, M., McCaffrey, P., Walser, E., Moen, S., Vo, A., & Ho, J. C. (2024). Machine-learning-enabled diagnostics with improved visualization of disease lesions in chest X-ray images. Diagnostics, 14(16), 1-17, Article 1699. https://doi.org/10.3390/diagnostics14161699
ORCID
0000-0002-2122-2572 (Pokojovy)
Repository Citation
Rahman, Md. Fashiar; Tseng, Tzu-Liang (Bill); Pokojovy, Michael; McCaffrey, Peter; Walser, Eric; Moen, Scott; Vo, Alex; and Ho, Johnny C., "Machine-Learning-Enabled Diagnostics with Improved Visualization of Disease Lesions in Chest X-Ray Images" (2024). Mathematics & Statistics Faculty Publications. 266.
https://digitalcommons.odu.edu/mathstat_fac_pubs/266
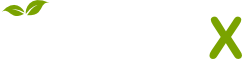
- Citations
- Citation Indexes: 2
- Usage
- Downloads: 27
- Abstract Views: 2
- Captures
- Readers: 13
- Mentions
- Blog Mentions: 1
- News Mentions: 1
Included in
Artificial Intelligence and Robotics Commons, Bacterial Infections and Mycoses Commons, Diagnosis Commons, Radiology Commons, Theory and Algorithms Commons