College
Frank Batten College of Engineering and Technology
Department
Electrical/Computer Engineering
Program
PhD Electrical & Computer Engineering
Publication Date
4-2021
DOI
10.25883/scba-hx03
Abstract
Clustering is widely used in unsupervised machine learning to partition a given set of data into non-overlapping groups. Many real-world applications require processing more complex multivariate time series data characterized by more than one dependent variables. A few works in literature reported multivariate classification using Shapelet learning. However, the clustering of multivariate time series signals using Shapelet learning has not explored yet. Shapelet learning is a process of discovering those Shapelets which contain the most informative features of the time series signal. Discovering suitable Shapelets from many candidates Shapelet has been broadly studied for classification and clustering of univariate time series signals. Shapelet learning has shown promising results in the case of univariate time series analysis. The analysis of multivariate time series signals is not widely explored because of the dimensionality issue. This work proposes a generalized Shapelet learning method for unsupervised multivariate time series clustering. The proposed method utilizes spectral clustering and Shapelet similarity minimization with least square regularization to obtain the optimal Shapelets for unsupervised clustering. The proposed method is evaluated using an in-house multivariate time series dataset on detection of radio frequency (RF) faults in the Jefferson Labs Continuous Beam Accelerator Facility (CEBAF). The dataset constitutes of three-dimensional time series recordings of three RF fault types. The proposed method shows successful clustering performance with average value of a precision of 0.732, recall of 0.717, F-score of 0.732, a rand index (RI) score of 0.812 and normalize mutual information (NMI) of 0.56 with overall less than 3% standard deviation in a five-fold cross validation evaluation.
Keywords
Shapelet, Multivariate
Disciplines
Electrical and Computer Engineering | Longitudinal Data Analysis and Time Series
Files
Download Full Text (372 KB)
Recommended Citation
Rahman, Md Monibor; Vidyaratne, Lasitha; Glandon, Alex; and Iftekharuddin, Khan, "Unsupervised Multivariate Time Series Clustering" (2021). College of Engineering & Technology (Batten) Posters. 5.
https://digitalcommons.odu.edu/gradposters2021_engineering/5
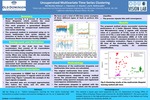
Included in
Electrical and Computer Engineering Commons, Longitudinal Data Analysis and Time Series Commons